- Machine Learning Times
- Posts
- The State of AI Report 2022 in Summary
The State of AI Report 2022 in Summary
Summary from The report by AI investors Nathan Benaich and Ian Hogarth.
The State of AI Report 2022 in Summary
Hey Guys,
Good morning to you, this is a summary In 4,800 words of one of the better reports on A.I. we have.
I wanted to consolidate my summaries so far on the topic of the State of AI Report in once place. Let's see if beehiive allows me to type more than Substack does, where images and links hit Gmail's limit really fast.
My Mission Statement
Covering A.I. also means for me now covering startups, papers, robotics trends, quantum computing and other areas of data science, the modern data stack, DevOps, MLOps and low and no-code solutions. My current feed lives here. This allows me to write about and save you time on all these incredible topics.
I write more than 16 posts a month on A.I., given that I have multiple Newsletters related to the topic at different intersections. To my knowledge, I'm one of the only analysts who does this. I'm saving Professionals time by curating, summarizing and distilling topic trends in artificial intelligence across society, business and the future.
My work is best appreciated by serious A.I. enthusiasts, those working in technology and News junkies. So typically that's what you are getting with my work. So let's get into it:
The State of AI in 2022
Summary from The report by AI investors Nathan Benaich and Ian Hogarth.
Artificial intelligence is complex and many sided. I could spend a lifetime blogging about it and barely scratch the surface. While I am naturally skeptical of reports by consulting firms or Venture Capitalists (no matter how versed in A.I), it’s always worth a look and a quick glance to see what they are saying.
I don’t always know what to make of these summaries, but there are some important conclusions, graphs, tidbits and insights of A.I.’s global nature and its commercial politics between public, private, national and industry groups. It’s surprisingly difficult to be objective about it all.
While AI’s growing impact on society and the economy is now evident, their report highlights that research into AI safety and the impact of AI still lags behind its rapid commercial, civil, and military deployment.
People tend to hype different aspects, while journalists are also looking to be read with sometimes suspect clickbait headlines. A lot of the report reads like praises for Google’s research. The bias here is definately a Venture Capital slant. This means it's also about hyping up A.I. as a field and its startups. Just keep that context in mind.
There are however some important trends that some might have considered unexpected in 2022. If you think about it, small, previously unknown labs like Stability.ai and Midjourney have developed text-to-image models of similar capability to those released by OpenAI and Google earlier in the year, and made them available to the public via API access and open sourcing.
Meanwhile, AI continues to advance scientific research.
Nathan Benaich’s own key takeaways are not those I would have chosen, but still let’s try to unpack their report:
Key takeaways
We hope the report has something for everyone- from AI research to politics. Here are five key findings:
APPLIED
1) AI is stepping up in more concrete ways: AI is increasingly being applied to mission critical infrastructure like national electric grids and automated supermarket warehousing calculations during pandemics.
BIOLOGY
2) AI-first approaches have taken biology by storm: AI has enabled faster simulations of humans’ cellular machinery (proteins and RNA) which has the potential to transform drug discovery and healthcare.
TRANSFORMERS
3) Transformers have emerged as a general purpose architecture for machine learning: beating the state of the art in many domains including NLP, computer vision, and even protein structure prediction.
VENTURE CAPITAL HYPE CONTINUES
4) Investors have taken notice: We have seen record funding this year into AI startups, and two first ever IPOs for AI-first drug discovery companies, as well as blockbuster IPOs for data infrastructure and cybersecurity companies that help enterprises retool for the AI-first era.
CHINA TOP IN RESEARCH AND PAPERS
5) China's ascension in research quality is notable: China’s universities have rocketed from publishing no AI research in 1980 to the largest volume of quality AI research today.
Read the State of AI Report
It’s also a bit fun that they attempt to make predictions and take a tally over their previous predictions. The report is 114 Google slides, so it’s not exactly exhaustive. They try to take the following into account as dimensions:
Research
Industry
Politics
Safety
Predictions
There was not much chatter on Hacker News or Reddit about the 2022 edition of this report. This is the 5th edition of the State of AI report, in 2022.
For additional summaries of this report, consider supporting me and the channel.
Subscribed
This is AI Supremacy Premium.
Black Box of Generative AI Unleashed
New research collectives have open sourced breakthrough AI models developed by large centralized labs at a never before seen pace. By contrast, the large-scale AI compute infrastructure that has enabled this acceleration, however, remains firmly concentrated in the hands of NVIDIA despite investments by Google, Amazon, Microsoft and a range of startups.
Executive Summary
Research
Diffusion models took the computer vision world by storm with impressive text-to-image generation capabilities.
AI attacks more science problems, ranging from plastic recycling, nuclear fusion reactor control, and natural product discovery.
Scaling laws refocus on data: perhaps model scale is not all that you need. Progress towards a single model to rule them all.
Community-driven open sourcing of large models happens at breakneck speed, empowering collectives to compete with large labs.
Inspired by neuroscience, AI research are starting to look like cognitive science in its approaches.
Industry
Have upstart AI semiconductor startups made a dent vs. NVIDIA? Usage statistics in AI research shows NVIDIA ahead by 20-100x.
Big tech companies expand their AI clouds and form large partnerships with A(G)I startups.
Hiring freezes and the disbanding of AI labs precipitates the formation of many startups from giants including DeepMind and OpenAI.
Major AI drug discovery companies have 18 clinical assets and the first CE mark is awarded for autonomous medical imaging diagnostics.
The latest in AI for code research is quickly translated by big tech and startups into commercial developer tools.
Politics
The chasm between academia and industry in large scale AI work is potentially beyond repair: almost 0% of work is done in academia.
Academia is passing the baton to decentralized research collectives funded by non-traditional sources.
The Great Reshoring of American semiconductor capabilities is kicked off in earnest, but geopolitical tensions are sky high.
AI continues to be infused into a greater number of defense product categories and defense AI startups receive even more funding.
Safety
AI Safety research is seeing increased awareness, talent, and funding, but is still far behind that of capabilities research.
State of AI: Evolution
AI research is moving so fast, it seems like almost every week there are new breakthroughs, with commercial applications quickly following suit. Case in point: AI coding assistants have been deployed, with early signs of developer productivity gains and satisfaction.
Papers and Research in A.I. is accelerating.
Decentralization Research Collectives and hubs like Hugging Face are inheriting many Academic projects.
AI is being used more in drug discovery and shows promise in genomics and biotechnology as well as broader applications in Healthcare as a whole.
AI in coding and software development is a key area of progress in 2022.
Their 2021 predictions vs. 2022 reality:

The Report gives a lot of credit to Google’s DeepMind in the life sciences.
Research: Reinforcement learning could be a core component of the next fusion breakthrough.
DeepMind’s Alpha Fold 2: AlphaFold mentions in AI research literature is growing massively and is predicted to triple year on year.
Genomics breakthroughs with A.I. poised for progress in 2020s: OpenCell: understanding protein localization with a little help from machine learning
Corporate AI labs rush into AI for code research
The report also gives a nod to Microsoft.
OpenAI’s Codex, which drives GitHub Copilot, has impressed the computer science community with its ability to complete code on multiple lines or directly from natural language instructions. This success spurred more research in this space, including from Salesforce, Google and DeepMind.
It’s hard to consider OpenAI a separate entity at this point. Not just Salesforce and Google though, Amazon and Meta.
Limitation of Transformers 2017-xyz
Are 5 years old but….The attention layer at the core of the transformer model famously suffers from a quadratic dependence on its input. A slew of papers promised to solve this, but no method has been adopted. SOTA LLMs come in different flavors (autoencoding, autoregressive, encoder-decoders), yet all rely on the same attention mechanism.
Language Models are good at Maths
Mathematical abilities of Language Models largely surpass expectations.
Built on Google’s 540B parameter LM PaLM, Google’s Minerva achieves a 50.3% score on the MATH benchmark (43.4 pct points better than previous SOTA), beating forecasters expectations for best score in 2022 (13%). Meanwhile, OpenAI trained a network to solve two mathematical olympiad problems (IMO).
Fast progress in LLM research renders benchmarks obsolete. Only 66% of machine learning benchmarks have received more than 3 results at different time points, and many are solved or saturated soon after their release.
If you need evidence of A.I’s rapid progress in 2022 I think this might be a good point: Rapid LLM progress and emerging capabilities seem to outrun current benchmarks.
LM Scaling Laws
DeepMind revisited LM scaling laws and found that current LMs are significantly undertrained: they’re not trained on enough data given their large size. They train Chinchilla, a 4x smaller version of their Gopher, on 4.6x more data, and find that Chinchilla outperforms Gopher and other large models on BIG-bench.

Emergence is not fully understood: it could be that for multi-step reasoning tasks, models need to be deeper to encode the reasoning steps. For memorization tasks, having more parameters is a natural solution. The metrics themselves may be part of the explanation, as an answer on a reasoning task is only considered correct if its conclusion is. Thus despite continuous improvements with model size, we only consider a model successful when increments accumulate past a certain point.
Next Frontiers
Language models can learn to use tools such as search engines and calculators, simply by making available text interfaces to these tools and training on a very small number of human demonstrations.
OpenAI’s WebGPT was the first model to demonstrate this convincingly by fine-tuning GPT-3 to interact with a search engine to provide answers grounded with references.
Adept, a new AGI company, is commercializing this paradigm. The company trains large transformer models to interact with websites, software applications and APIs. (The Report mentioned Adept AI many times, likely a sponsored product placement).
Compute in Machine Learning (Contemporary Eras)
The Pre-Deep Learning Era (pre-2010, training compute doubled every 20 months), the Deep Learning Era (2010-15, doubling every 6 months), and the Large-Scale Era (2016-present, a 100-1000x jump, then doubling every 10 months).
Before 2010: - time to double = 20 months.
Deep Learning Era 2010-2015 - time to double = 6 months.
Large-Scale Era 2016-2023 - a 100-1000x jump, time to double = 6 months.
So here is yet another empirical way to measure A.I’s progress as accelerating post 2018 or so.

New Dominance of Diffusion Models
Diffusion models take over text-to-image generation and expand into other modalities.
Diffusion models (DMs) learn to reverse successive noise additions to images by modeling the inverse distribution (generating denoised images from noisy ones) at each step as a Gaussian whose mean and covariance are parametrized as a neural network. DMs generate new images from random noise.
SOTA text-to-image models like DALL-E 2, Imagen and Stable Diffusion are based on DMs.
Midjourney is reportedly profitable, and Stability has already open-sourced their model.
Research on diffusion-based text-to-video generation was kicked-off around April 2022, with work from Google and the University of British Columbia. But in late September, new research from Meta and Google came with a jump in quality, announcing a sooner-than-expected DALL-E moment for text-to-video generation.
Like I have mentioned I actually consider Phenaki the front runner here. C-ViViT is an encoder-decoder structure with unique capabilities. It can exploit temporal information in videos by compressing them in temporal and spatial dimensions while staying auto-regressive in time.
GPT-3 is set to Retire Soon

I think we can expect GPT-4 to arrive fairly soon (as of October, 2022). GPT-3 clearly is a foundational model. GPT stands for Generative Pre-trained Transformer. As far as I know, there's little public info about GPT-4: what it'll be like, its features, or its abilities.
DALL-E 2 Also Spawned some Good Progress

How useful are LLMs?
LLMs empower robots to execute diverse and ambiguous instructions
In the early 2020s Transformers are becoming truly cross-modality
Dangers of AI in Drug Discovery?
Researchers from Collaborations Pharmaceuticals and King’s College London showed that machine learning models designed for therapeutic use can be easily repurposed to generate biochemical weapons.
So this goes up to about page 47 in summary of the report, so a little less than half way. If you want me to summarize the other half, do let me know or read the report yourself.
End of Part I
This is a continuation of my summary of the State of AI Report, 5th edition, 2022. You can see the first half here.
On October 11, 2022, the 113-slide, open-access State of AI Report 2022 was released. It reveals many of the topics we cover here on A.I. Supremacy, check our archives.
I want to note that Deloitte’s own State of AI report also fifth edition made some interesting headlines:
For Deloitte’s “State of AI in the Enterprise,” Fifth Edition, we surveyed 2,620 global business leaders representing six industry areas and dozens of sectors. Key findings include:
Deloitte’s own A.I. Key Takeaways
Ninety-four (94%) percent of business leaders surveyed agree that AI is critical to success over the next five years.
Seventy-nine (79%) percent of leaders say they have fully deployed three or more AI applications, compared to 62% last year.
There was a 29% increase in the number of respondents self-identifying as “underachievers,” suggesting that many organizations are struggling to achieve meaningful AI outcomes.
Top challenges associated with scaling according to respondents are managing AI-related risk (50%), lack of executive commitment (50%), lack of maintenance and post launch support (50%).
This indicates that in business A.I. adoption is strong and awareness is also high, even with some lack of executive commitment still holding things back.
Now we return to regular programming.
Credits
Detailing the exponential progress in the field of AI and focusing on developments since last year’s edition, the report was authored by Nathan Benaich, General Partner, Air Street Capital; Ian Hogarth, Plural Platform cofounder; Othmane Sebbouh, machine learning PhD student, ENS Paris, CREST-ENSAE, CNRS; and Nitarshan Rajkumar, PhD student in AI.
Benaich is the general partner of Air Street Capital, a venture capital firm investing in Al-first technology and life science companies.
Is China Leading ‘Surveillance Capitalism’?
What Google started, China seems to be finishing better, at least China’s academic papers would seem to indicate this.
Compared to US AI research, Chinese papers focus more on surveillance related-tasks. These include autonomy, object detection, tracking, scene understanding, action and speaker recognition.
This bias of focus of facial recognition and image recognition will mean China will be better at certain things.
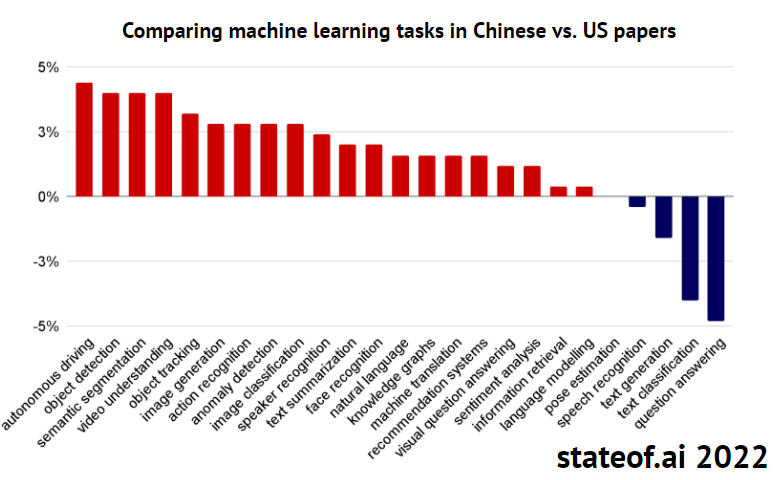
Autonomous Driving
Objective Recognition
Image recognition
Anomaly detection
Text summarization
Face Recognition
Natural language
So what does this suggest?
The top academic countries in number of papers in A.I. are:

United States
China
United Kingdom
Germany
France
Canada
Just as China’s economy and technology sector may catch and overtake the U.S. in the 2030s, it’s been suggested that this is or will be the case in A.I. papers and engineering.
While US-based authors published more AI papers than Chinese peers in 2022, China and Chinese institutions are growing their output at a faster rate.
By what year will China definitively overtake the U.S. in A.I. Research?
Some have suggested in 2022 it has already occurred, conservatively I think by 2032 there it will have easily.
Curiously in the Fall of 2022, Chinese stocks have tumbled in value in the American stock market, far lower than their real intrinsic value would seem to indicate. This could be due to risks of de-listing, political and sanctions like recently in A.I. chips and semiconductor parts. Curiously, Taiwan (TSMC) and South Korean companies have gotten a one year exemption from these bans.
Major Pillars of A.I. Hype and Changes in 2022
Coding Assistants
Text to Image Generation (Text to Art)
Distributed Research Collectives (more decentralized A.I. Labs that partner with BigTech)
Nvidia still A.I. Hardware leader
Semiconductor geopolitical dislocation
Major academic papers and MLops companies accelerating
Clumsy and regionally disorganized state of AI Safety

Read AI Supremacy in the Substack appAvailable for iOS and AndroidGet the app
Some of the confusion between Chinese and U.S. paper indexes in A.I. has to do with language. The China-US AI research paper gap explodes if we include the Chinese-language database, China National Knowledge Infrastructure.
Upstart A.I. Chip Companies are Coming

There are literally dozens of A.I. chip companies in the U.S. and China that have an off-chance of becoming important in the late 2020s and 2030s.
NVIDIA has over 3 million developers on their platform and the company’s latest H100 chip generation is expected to deliver 9x training performance vs. the A100. Meanwhile, revenue figures for Cerebras, SambaNova and Graphcore are not publicly available.
BigTech is Aligning So called A.I. Labs

Basically buying them off.
Inflection and Adept.AI have yet to have BigTech masters.
Even as leading Cloud providers adopt Quantum computing vendors, they also race to build their own Supercomputers. In a gold rush for compute, companies build bigger than national supercomputers. Some believe this will give them some edge in A.I. (e.g. Meta) or autonomous driving (e.g. Tesla).
The Decentralized Movement in the Democratization of A.I.
2022 also saw a major movement of DeepMind (and Google Brain) and OpenAI alums forming new startups and Meta disbands its (“centralized”) core AI group.
This spawned more energy for Hugging Face and others:
Birth of new startups and more decentralized AI Labs:

DeepMind and OpenAI for all the good work they do and costly integrations with Alphabet and Microsoft, they motivated new companies and new ways of doing things.

The New Breed of AGI Startups

Anthropic
Inflection
Co:here
Adept
Here our coverage once again ends on slide 61 of 113 of the State of AI Report 2022.
End of Part II
I’ve been summarizing The State of AI Report thus far in two posts, here and here. Here we continue that discussion.
UK Universities are some of the best in artificial intelligence and spin-outs from academia into startups are quite common.
Universities are an important source of AI companies including Databricks, Snorkel, SambaNova, Exscientia and more. In the UK, 4.3% of UK AI companies are university spinouts, compared to 0.03% for all UK companies. AI is indeed among the most represented sectors for spinouts formation. But this comes at a steep price: Technology Transfer Offices (TTOs) often negotiate spinout deal terms which are unfavorable to founders, e.g. a high equity share in the company or royalties on sales.

Meanwhile in A.I in healthcare, AI in pharma, AI in early diagnosis (imaging) and surgical robots are evolving quickly.
Lithuanian startup Oxipit received the industry’s first autonomous certification for their computer vision-based diagnostic. The system autonomously reports on chest X-rays that feature no abnormalities, removing the need for radiologists to look at them.
With aging populations there’s a huge crisis of healthcare staff shortages comes. For instance Japan thinks it needs 1 million more healthcare workers to cope. Medical profession’s pandemic burnout, falling birth rate, fewer young people working, and longer lives have brought and will bring intense pressure that may accelerate A.I.’s adoption in healthcare in many ways.
Venture Capital Slowdown of 2022 and 2023 is Real
In 2022, investment in startups using AI has slowed down along with the broader market. However the boom of 2020 and 2021 likely will make up for it according to the latest data.

Source: State of AI Report 2022.
Funding is down about 36% in 2022. But that’s from a very strong year of 2021.
Since around 2018, funding for A.I. has increased significantly globally.
Hyper-funding of A.I. has only occurred since about 2015. Even as self-driving cars have spent around $75-100 Billion without robo-taxis as scale, by 2030 we’ll know more about the future of A.I. to make better guesses of its overall impact in the 21st century.
In 2022 and 2023 there will be less mega-rounds in A.I, like the kind we might have seen with Softbank.
The drop in VC investment is most noticeable in 100M+ rounds, whereas smaller rounds are expected to amount to $30.9B worldwide by the end of 2022, which is almost on track with the 2021 level. This is normal with higher interest rates and less access to free cash in a changed macro environment.
The Startup Valuation Reset of 2022
Public valuations have dropped dramatically in 2022. Even many public companies that are growth stocks are down 50-75% so far in 2022. It makes one question how OpenAI thinks its valuation is nearly $20 Billion (WSJ).
U.S. Still Leads in A.I. Unicorns
The US leads by the number of AI unicorns, followed by China & the UK. Even as China is supposedly very strong in research and A.I. papers that hasn’t yet translated into viable A.I. Unicorns at scale.

According to the data, the U.S. still has over 4x more A.I. Unicorns than China, with over 3x the economic enterprise value.
So by this metric, the U.S. is still far ahead of China in terms of A.I. development.
Outside of the U.S. and China, the other countries on the list bring a nearly insignificant number of A.I. Unicorns including:
UK
Israel (impressive per Capita)
Germany
Canada
Singapore (impressive per Capita)
Switzerland
So basically the entire fate of commercial and enterprise A.I. is in the hands of the U.S. and China for the most part which results in highly centralized BigTech A.I. Supremacy. Of course for safety and A.I ethics, this is not good.
The problem is these numbers are deceptive, as ByteDance’s marketing spend as an A.I. consumer apps company is not factored in to numbers like these:

TikTok is recently claiming it’s an Entertainment company, and not even a competitor of Instagram, which is pretty absurd. This all indicates China is getting better as Silicon Valley’s innovation is slowing down even at the highest levels of BigTech, with the valuation crunch of Facebook, which now refers to itself as “Meta”.
Enterprise software is the most invested category globally, while robotics captures the largest share of VC investment into AI. Yet things like robotics, robo-taxis, quantum computing and A.I. in healthcare are all just nascent stage of development and may remain so for many years to come. Thus the A.I. maturity of companies in the mainstream may actually occur more in the 2030s and 2040s rather than the nascent 2020s. That functionally gives China a lot of time to catch up in the semiconductor supply chain and Cloud computing space, even as it is ahead in mobile app innovation and E-commerce.
Biggest Categories of AI Funding
Enterprise software is the most invested category globally, while robotics captures the largest share of VC investment into AI.

Enterprise Software
Transportation
Fintech
Healthcare
Robotics
Food
Marketing
Security
Media
Telecom
Semiconductors
Education
Energy
Travel
Real Estate
Gaming
Home living
Jobs recruitment
Legal
Sports
Music
Fashion
Hosting
Wellness & Beauty
Event Tech
Kids
Dating
So clearly A.I. funding and A.I. startups have quite a wide range which is interesting to know by the data.
If IPOs and SPACs in 2022 declined sharply from their 2021 high, M&A is up a lot in 2022. This is especially true in 2022 for the Eurozone, notably in Scandinavia.
Politics and A.I.
With chip bans the U.S. and China are truly in an economic war for the future of artificial intelligence. Just today Chinese stocks plunged with the new political leadership announced in China, basically supporting the status quo who are not perceived as Chinese BigTech friendly due to their obsession with “common prosperity” element of Socialism with Chinese characteristics.
Corporations and Accessibility
Large scale A.I. also has more intense requirements. The compute requirements for large-scale AI experiments has increased >300,000x in the last decade.
So BigTech doesn’t just win in talent, it wins in compute because Academics can’t keep up in such a world. Over the same period, the % of these projects run by academics has plummeted from ~60% to almost 0%. If the AI community is to continue scaling models, this chasm of “have” and “have nots” creates significant challenges for AI safety, pursuing diverse ideas, talent concentration, and more.
This is pretty shocking and not readily understood by the general public.
Rise of Research Collectives
In 2022, there has been a rise of more decentralized “Research Collectives”.
March, 2022: Eleuther.ai
July, 2022: Big Science (Bloom)
August, 2022: Stability.AI
Hugging Face has been involved to some degree in many of these with dedicated pages and collaboration. Essentially slow progress in providing academics with more compute leaves others to act faster.
Clearly in 2023 this moment of decentralized research collectives (DRCs) will continue to proliferate to actually make A.I. more democratized and accessible, something BigTech claims to do but doesn’t really act upon very noticeably.
The State of AI Report 2022 goes so far as to suggest that The baton is passing from academia to decentralized research collectives.
We have arrived now at slide 84/113 of the report. My coverage will continue in future posts.
Part IV will continue in a future post.
Thanks for reading or scanning this summary. I'm passionate about A.I. coverage and work diligently to improve it as best I can learning along with you about the topic.
Why I write Newsletters about the Future
Did you enjoy this article? Give me a comment or send me a note. Or just hit REPLY to this Email. I may use this Newsletter to summarize some of the other work that I am up to on a day-to-day basis when I don't have Op-Eds that are top of mind to share. I really respect both beehiive and substack's approaches to sharing with a community and I want to be recognized as a major A.I. curator on both platforms.
That being said, I'm interested in web views as I do not find Email to be the best means of transmission. That's why I keep an active LinkedIn, with one of the top Newsletters on that platform here. However due to the lack of analytics on LinkedIn, I had to diversify across different Newsletter platforms. I'm still very unclear as to which is the best route to take.
As a Futurist, I want to give serious coverage of the future of technology. At the end of the day I'm not doing this for views, attention, recognition or monetization. I'm doing it because I find the niche valuable and all-encompassing, it allows me to write about startups, venture capital, geopolitics and nascent technology in a way I find extremely gratifyingly. I hope you find some of the topics gratifying and fascinating as well.