- Machine Learning Times
- Posts
- The State of A.I. According to Stanford's Human-Centered A.I. 2023 Index
The State of A.I. According to Stanford's Human-Centered A.I. 2023 Index
Some interesting infographics here.

Hey Everyone,
What can we learn from the recent State of AI 2023 report. Throughout the year there are important summaries of the State of A.I. I follow. Abhi Gupta made a concise summary of it in a LinkedIn post.
So the 2023 Artificial Intelligence (AI) Index Report Published by Stanford Institute for Human-Centered Artificial Intelligence (HAI) was just released on April 3rd, 2023.
This being said there are few A.I. Newsletters on Subsack I respect more than the AI Ethics Brief (who write rather long reports).
This post is long and may get clipped by Google. If that is the case for you, just read it online on the web view here. This post is about 5,000 words.

Also you can subscribe to the Responsible A.I. Bulletin on LinkedIn. In this post I’ll go into greater detail on the 2023 AI Index Report by the HAI. To know more about the AI ethics folk in Montreal, please visit: https://montrealethics.ai/about
TL;DR
Taking Abhi’s notes on his LinkedIn post, we can see how interest and worries about A.I. are increasing:
🧠 Industry races ahead of academia.
📔 Performance saturation on traditional benchmarks.
🌎 AI is both helping and harming the environment.
👩🔬The world’s best new scientist … AI?
😈 The number of incidents concerning the misuse of AI is rapidly rising.
📶The demand for AI-related professional skills is increasing across virtually every American industrial sector.
💰For the first time in the last decade, year-over-year private investment in AI decreased.
🚣While the proportion of companies adopting AI has plateaued, the companies that have adopted AI continue to pull ahead.
📄Policymaker interest in AI is on the rise.
His notes on LinkedIn are just highlights of the report.
The HAI asks:
Just like the earlier “State of AI Report”, the AI Index Report tracks, collates, distills, and visualizes data related to artificial intelligence.
So let’s get into it:

The Stanford HAI Artificial Intelligence Index Report 2023 was recently released. I wanted to share their highlights in a single document Email to you so you could slowly and easily digest some of their findings.
You can download the full report here. So these are their executive insights of each chapter. So read slowly and carefully for a great macro-perspective on the State of AI in 2023.
The AI Index is an independent initiative at the Stanford Institute for Human-Centered Artificial Intelligence (HAI), led by the AI Index Steering Committee, an interdisciplinary group of experts from across academia and industry.
Are you excited about the state of A.I. or another state? |
Report Highlights
Chapter 1: Research and Development
The United States and China had the greatest number of cross-country collaborations in AI publications from 2010 to 2021, although the pace of collaboration has slowed. The number of AI research collaborations between the United States and China increased roughly 4 times since 2010, and was 2.5 times greater than the collaboration totals of the next nearest country pair, the United Kingdom and China. However the total number of U.S.-China collaborations only increased by 2.1% from 2020 to 2021, the smallest year-over-year growth rate since 2010.
AI research is on the rise, across the board. The total number of AI publications has more than doubled since 2010. The specific AI topics that continue dominating research include pattern recognition, machine learning, and computer vision.
China continues to lead in total AI journal, conference, and repository publications. The United States is still ahead in terms of AI conference and repository citations, but those leads are slowly eroding. Still, the majority of the world’s large language and multimodal models (54% in 2022) are produced by American institutions.
Industry races ahead of academia. Until 2014, most significant machine learning models were released by academia. Since then, industry has taken over. In 2022, there were 32 significant industry-produced machine learning models compared to just three produced by academia. Building state-of-the-art AI systems increasingly requires large amounts of data, computer power, and money—resources that industry actors inherently possess in greater amounts compared to nonprofits and academia.
Large language models are getting bigger and more expensive. GPT-2, released in 2019, considered by many to be the first large language model, had 1.5 billion parameters and cost an estimated $50,000 USD to train. PaLM, one of the flagship large language models launched in 2022, had 540 billion parameters and cost an estimated $8 million USD—PaLM was around 360 times larger than GPT-2 and cost 160 times more. It’s not just PaLM: Across the board, large language and multimodal models are becoming larger and pricier.
Chapter 2: Technical Performance Performance saturation on traditional benchmarks.
Performance saturation on traditional benchmarks. AI continued to post state-of-the-art results, but year-over-year improvement on many benchmarks continues to be marginal. Moreover, the speed at which benchmark saturation is being reached is increasing. However, new, more comprehensive benchmarking suites such as BIG-bench and HELM are being released.
Generative AI breaks into the public consciousness. 2022 saw the release of text-to-image models like DALL-E 2 and Stable Diffusion, text-to-video systems like Make-A-Video, and chatbots like ChatGPT. Still, these systems can be prone to hallucination, confidently outputting incoherent or untrue responses, making it hard to rely on them for critical applications.
AI systems become more flexible. Traditionally AI systems have performed well on narrow tasks but have struggled across broader tasks. Recently released models challenge that trend; BEiT-3, PaLI, and Gato, among others, are single AI systems increasingly capable of navigating multiple tasks (for example, vision, language).
Capable language models still struggle with reasoning. Language models continued to improve their generative capabilities, but new research suggests that they still struggle with complex planning tasks.
AI is both helping and harming the environment. New research suggests that AI systems can have serious environmental impacts. According to Luccioni et al., 2022, BLOOM’s training run emitted 25 times more carbon than a single air traveler on a one-way trip from New York to San Francisco. Still, new reinforcement learning models like BCOOLER show that AI systems can be used to optimize energy usage
The world’s best new scientist … AI? AI models are starting to rapidly accelerate scientific progress and in 2022 were used to aid hydrogen fusion, improve the efficiency of matrix manipulation, and generate new antibodies.
AI starts to build better AI. Nvidia used an AI reinforcement learning agent to improve the design of the chips that power AI systems. Similarly, Google recently used one of its language models, PaLM, to suggest ways to improve the very same model. Self-improving AI learning will accelerate AI progress.
Chapter 3: Technical AI Ethics
The effects of model scale on bias and toxicity are confounded by training data and mitigation methods. In the past year, several institutions have built their own large models trained on proprietary data—and while large models are still toxic and biased, new evidence suggests that these issues can be somewhat mitigated after training larger models with instruction-tuning.
Generative models have arrived and so have their ethical problems. In 2022, generative models became part of the zeitgeist. These models are capable but also come with ethical challenges. Text-to-image generators are routinely biased along gender dimensions, and chatbots like ChatGPT can be tricked into serving nefarious aims.
The number of incidents concerning the misuse of AI is rapidly rising. According to the AIAAIC database, which tracks incidents related to the ethical misuse of AI, the number of AI incidents and controversies has increased 26 times since 2012. Some notable incidents in 2022 included a deepfake video of Ukrainian President Volodymyr Zelenskyy surrendering and U.S. prisons using call-monitoring technology on their inmates. This growth is evidence of both greater use of AI technologies and awareness of misuse possibilities.
Fairer models may not be less biased. Extensive analysis of language models suggests that while there is a clear correlation between performance and fairness, fairness and bias can be at odds: Language models which perform better on certain fairness benchmarks tend to have worse gender bias.
Interest in AI ethics continues to skyrocket. The number of accepted submissions to FAccT, a leading AI ethics conference, has more than doubled since 2021 and increased by a factor of 10 since 2018. 2022 also saw more submissions than ever from industry actors.
Automated fact-checking with natural language processing isn’t so straightforward after all. While several benchmarks have been developed for automated fact-checking, researchers find that 11 of 16 of such datasets rely on evidence “leaked” from fact-checking reports which did not exist at the time of the claim surfacing.
Chapter 4: The Economy
The demand for AI-related professional skills is increasing across virtually every American industrial sector. Across every sector in the United States for which there is data (with the exception of agriculture, forestry, fishing, and hunting), the number of AI-related job postings has increased on average from 1.7% in 2021 to 1.9% in 2022. Employers in the United States are increasingly looking for workers with AI-related skills.
For the first time in the last decade, year-over-year private investment in AI decreased. Global AI private investment was $91.9 billion in 2022, which represented a 26.7% decrease since 2021. The total number of AI-related funding events as well as the number of newly funded AI companies likewise decreased. Still, during the last decade as a whole, AI investment has significantly increased. In 2022 the amount of private investment in AI was 18 times greater than it was in 2013.
Once again, the United States leads in investment in AI. The U.S. led the world in terms of total amount of AI private investment. In 2022, the $47.4 billion invested in the U.S. was roughly 3.5 times the amount invested in the next highest country, China ($13.4 billion). The U.S. also continues to lead in terms of total number of newly funded AI companies, seeing 1.9 times more than the European Union and the United Kingdom combined, and 3.4 times more than China.
In 2022, the AI focus area with the most investment was medical and healthcare ($6.1 billion); followed by data management, processing, and cloud ($5.9 billion); and Fintech ($5.5 billion). However, mirroring the broader trend in AI private investment, most AI focus areas saw less investment in 2022 than in 2021. In the last year, the three largest AI private investment events were: (1) a $2.5 billion funding event for GAC Aion New Energy Automobile, a Chinese manufacturer of electric vehicles; (2) a $1.5 billion Series E funding round for Anduril Industries, a U.S. defense products company that builds technology for military agencies and border surveillance; and (3) a $1.2 billion investment in Celonis, a business-data consulting company based in Germany
While the proportion of companies adopting AI has plateaued, the companies that have adopted AI continue to pull ahead. The proportion of companies adopting AI in 2022 has more than doubled since 2017, though it has plateaued in recent years between 50% and 60%, according to the results of McKinsey’s annual research survey. Organizations that have adopted AI report realizing meaningful cost decreases and revenue increases.
Chapter 4: The Economy (cont’d)
AI is being deployed by businesses in multifaceted ways. The AI capabilities most likely to have been embedded in businesses include robotic process automation (39%), computer vision (34%), NL text understanding (33%), and virtual agents (33%). Moreover, the most commonly adopted AI use case in 2022 was service operations optimization (24%), followed by the creation of new AI-based products (20%), customer segmentation (19%), customer service analytics (19%), and new AI-based enhancement of products (19%).
AI tools like Copilot are tangibly helping workers. Results of a GitHub survey on the use of Copilot, a text-to-code AI system, find that 88% of surveyed respondents feel more productive when using the system, 74% feel they are able to focus on more satisfying work, and 88% feel they are able to complete tasks more quickly.
China dominates industrial robot installations. In 2013, China overtook Japan as the nation installing the most industrial robots. Since then, the gap between the total number of industrial robots installed by China and the next-nearest nation has widened. In 2021, China installed more industrial robots than the rest of the world combined.
Chapter 5: Education
More and more AI specialization. The proportion of new computer science PhD graduates from U.S. universities who specialized in AI jumped to 19.1% in 2021, from 14.9% in 2020 and 10.2% in 2010.
New AI PhDs increasingly head to industry. In 2011, roughly the same proportion of new AI PhD graduates took jobs in industry (40.9%) as opposed to academia (41.6%). Since then, however, a majority of AI PhDs have headed to industry. In 2021, 65.4% of AI PhDs took jobs in industry, more than double the 28.2% who took jobs in academia.
New North American CS, CE, and information faculty hires stayed flat. In the last decade, the total number of new North American computer science (CS), computer engineering (CE), and information faculty hires has decreased: There were 710 total hires in 2021 compared to 733 in 2012. Similarly, the total number of tenure-track hires peaked in 2019 at 422 and then dropped to 324 in 2021.
The gap in external research funding for private versus public American CS departments continues to widen. In 2011, the median amount of total expenditure from external sources for computing research was roughly the same for private and public CS departments in the United States. Since then, the gap has widened, with private U.S. CS departments receiving millions more in additional funding than public universities. In 2021, the median expenditure for private universities was $9.7 million, compared to $5.7 million for public universities.
Interest in K–12 AI and computer science education grows in both the United States and the rest of the world. In 2021, a total of 181,040 AP computer science exams were taken by American students, a 1.0% increase from the previous year. Since 2007, the number of AP computer science exams has increased ninefold. As of 2021, 11 countries, including Belgium, China, and South Korea, have officially endorsed and implemented a K–12 AI curriculum.
Chapter 6: Policy and Governance
Policymaker interest in AI is on the rise. An AI Index analysis of the legislative records of 127 countries shows that the number of bills containing “artificial intelligence” that were passed into law grew from just 1 in 2016 to 37 in 2022. An analysis of the parliamentary records on AI in 81 countries likewise shows that mentions of AI in global legislative proceedings have increased nearly 6.5 times since 2016.
From talk to enactment—the U.S. passed more AI bills than ever before. In 2021, only 2% of all federal AI bills in the United States were passed into law. This number jumped to 10% in 2022. Similarly, last year 35% of all state-level AI bills were passed into law.
When it comes to AI, policymakers have a lot of thoughts. A qualitative analysis of the parliamentary proceedings of a diverse group of nations reveals that policymakers think about AI from a wide range of perspectives. For example, in 2022, legislators in the United Kingdom discussed the risks of AI-led automation; those in Japan considered the necessity of safeguarding human rights in the face of AI; and those in Zambia looked at the possibility of using AI for weather forecasting.
The U.S. government continues to increase spending on AI. Since 2017, the amount of U.S. government AI-related contract spending has increased roughly 2.5 times.
The legal world is waking up to AI. In 2022, there were 110 AI-related legal cases in United States state and federal courts, roughly seven times more than in 2016. The majority of these cases originated in California, New York, and Illinois, and concerned issues relating to civil, intellectual property, and contract law.
Chapter 7: Diversity
North American bachelor’s, master’s, and PhD-level computer science students are becoming more ethnically diverse. Although white students are still the most represented ethnicity among new resident bachelor’s, master’s, and PhD-level computer science graduates, students from other ethnic backgrounds (for example, Asian, Hispanic, and Black or African American) are becoming increasingly more represented. For example, in 2011, 71.9% of new resident CS bachelor’s graduates were white. In 2021, that number dropped to 46.7%.
New AI PhDs are still overwhelmingly male. In 2021, 78.7% of new AI PhDs were male. Only 21.3% were female, a 3.2 percentage point increase from 2011. There continues to be a gender imbalance in higher-level AI education.
Women make up an increasingly greater share of CS, CE, and information faculty hires. Since 2017, the proportion of new female CS, CE, and information faculty hires has increased from 24.9% to 30.2%. Still, most CS, CE, and information faculty in North American universities are male (75.9%). As of 2021, only 0.1% of CS, CE, and information faculty identify as nonbinary.
American K–12 computer science education has become more diverse, in terms of both gender and ethnicity. The share of AP computer science exams taken by female students increased from 16.8% in 2007 to 30.6% in 2021. Year over year, the share of Asian, Hispanic/Latino/Latina, and Black/African American students taking AP computer science has likewise increased.
Does anything stand out to you?
Chapter 8: Public Opinion
Chinese citizens are among those who feel the most positively about AI products and services. Americans … not so much. In a 2022 IPSOS survey, 78% of Chinese respondents (the highest proportion of surveyed countries) agreed with the statement that products and services using AI have more benefits than drawbacks. After Chinese respondents, those from Saudi Arabia (76%) and India (71%) felt the most positive about AI products. Only 35% of sampled Americans (among the lowest of surveyed countries) agreed that products and services using AI had more benefits than drawbacks.
Men tend to feel more positively about AI products and services than women. Men are also more likely than women to believe that AI will mostly help rather than harm. According to the 2022 IPSOS survey, men are more likely than women to report that AI products and services make their lives easier, trust companies that use AI, and feel that AI products and services have more benefits than drawbacks. A 2021 survey by Gallup and Lloyd’s Register Foundation likewise revealed that men are more likely than women to agree with the statement that AI will mostly help rather than harm their country in the next 20 years.
People across the world and especially America remain unconvinced by self-driving cars. In a global survey, only 27% of respondents reported feeling safe in a self-driving car. Similarly, Pew Research suggests that only 26% of Americans feel that driverless passenger vehicles are a good idea for society.
Different causes for excitement and concern. Among a sample of surveyed Americans, those who report feeling excited about AI are most excited about the potential to make life and society better (31%) and to save time and make things more efficient (13%). Those who report feeling more concerned worry about the loss of human jobs (19%); surveillance, hacking, and digital privacy (16%); and the lack of human connection (12%).
NLP researchers … have some strong opinions as well. According to a survey widely distributed to NLP researchers, 77% either agreed or weakly agreed that private AI firms have too much influence, 41% said that NLP should be regulated, and 73% felt that AI could soon lead to revolutionary societal change. These were some of the many strong opinions held by the NLP research community.
What followers are some of the infographics of the report that stood out to me:
Number of A.I. Publications Globally 2010 to 2021
Noticeable acceleration since 2018.

AI Journal Publications by Geographical Area 2010-21

Top Ten Institutions in the World in 2021 Ranked by Number of AI Publication in All Fields, 2010-21

Top Ten Institutions in the World by Number of AI Publications in All Fields Continued, 2010-21

Shocking how dominant China is in A.I. publications and research in academic institutions (if the research is legit, some believe some of them are faked).
Thanks for reading!
Artificial Intelligence Survey 🤖🏦🧭 is a reader-supported publication. To receive new posts and support my work, consider becoming a free or paid subscriber.
I’m obsessed with the new A.I. report by the HAI.
Get the latest insights and links on a feed tagged with human feedback in A.I. here.
The Stanford HAI Artificial Intelligence Index Report 2023 was recently released. I wanted to share their highlights in a single Email with visuals for you, so you could slowly and easily digest some of their findings.
You can download the full report here.
Number of A.I. Publications Globally 2010 to 2021
Noticeable acceleration since 2018.

AI Journal Publications by Geographical Area 2010-21

Top Ten Institutions in the World in 2021 Ranked by Number of AI Publication in All Fields, 2010-21

Top Ten Institutions in the World by Number of AI Publications in All Fields Continued, 2010-21
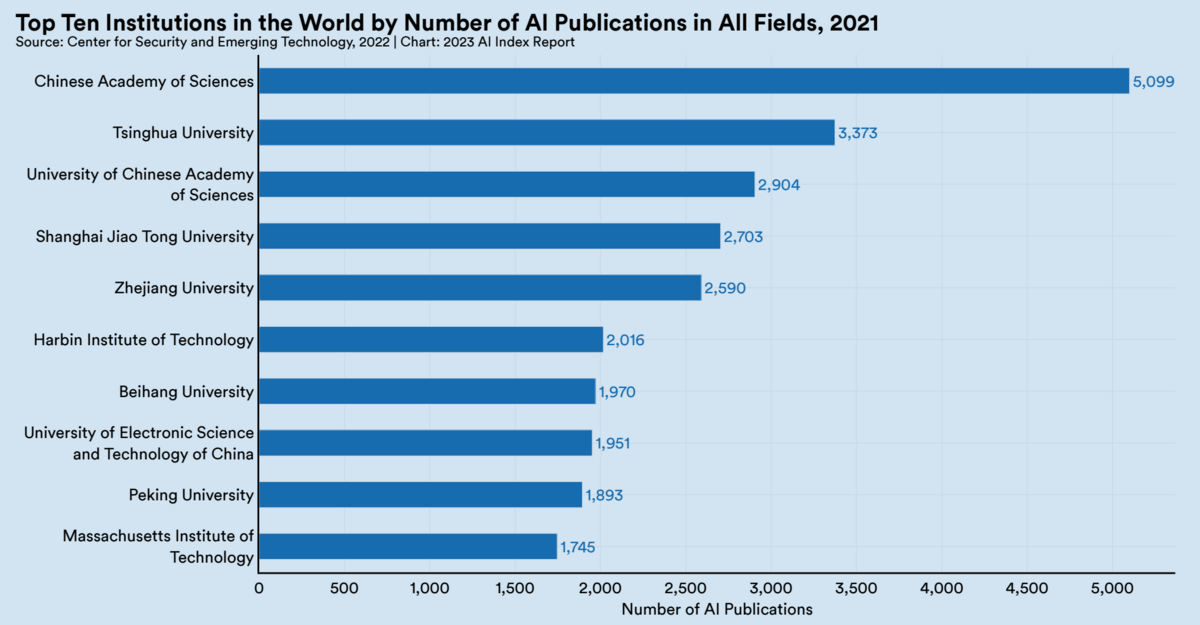
Estimated Training Costs of LLMs that are Scaling up
Between 2019 and 2023 we went from LLMs of 1.5 Billion parametres to LLMs of 1 Trillion parametres.

Shana Lynch writes:
Countries with the Most Favorable view of A.I. in terms of Public Opinion

There is a Substantial Increase in A.I. Labor Demand

HAI writes:
Countries with the Lowest Trust and Least Favorable View of A.I. Products
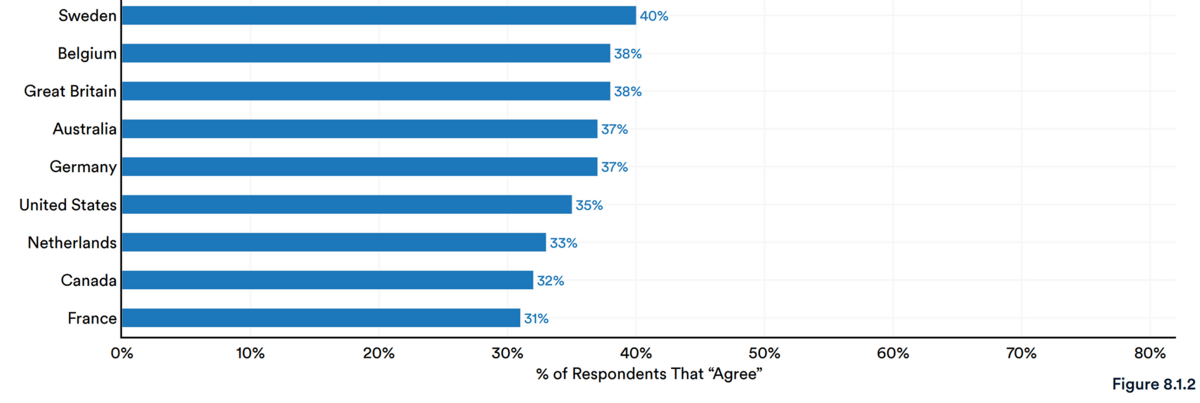
Why is that?
Which Countries Lead in Industrial Robots?

China
Japan
United States
South Korea
Germany
Italy
Taiwan
(Now imagine than in terms of GDP, per capita or human jobs to robot jobs).
South Korea
Taiwan
Canada
Singapore take on a new light.
Corporate Investment in A.I. actually Dipped in 2022

HAI Wrote:
In a single decade, global corporate investment in AI increased 13x.
Microsoft with Nuance and OpenAI truly lead BigTech investment in recent times.
Where is Adoption of Industrial Robots Occuring the Fastest?

Meanwhile China has more industrial robot adoption than the rest of the world combined.
PhDs in A.I. are Rushing to Industry

HAI Notes:
Download the PDF to comb through the data yourself.
U.S. Federal Budget for A.I. Increases YOY

The Wild Wild West Period of A.I. 2021 to 2026

“Artificial Intelligence has the potential to help us realize our shared dream of a better future for all of humanity. At Stanford HAI, our vision is led by our commitment to studying, guiding and developing human-centered AI technologies and applications.”
- John Etchemendy & Fei-Fei Li, Denning Co-Directors
We are on the cusp of The Age of Artificial Intelligence.
The AI Index is an independent initiative at the Stanford Institute for Human-Centered Artificial Intelligence (HAI), led by the AI Index Steering Committee, an interdisciplinary group of experts from across academia and industry.
The annual report tracks, collates, distills, and visualizes data relating to artificial intelligence, enabling decision-makers to take meaningful action to advance AI responsibly and ethically with humans in mind.
Direct to Full Text Report (HTML Version)
Direct to Full Text Report (386 pages; PDF)
Direct to Public Data File
Direct to Complete Publication Announcement (Includes Letter For Co-Directors of HAI)
Executive Summary

Large language models keep scaling in size and expense. GPT-2, released in 2019 and considered the first large language model, had 1.5 billion parameters and cost an estimated $50,000 to train. Just three years later, PaLM launched with 540 billion parameters and cost an estimated $8 million. GPT-4 is believed to have 1 Trillion parameters, according to a report by Semafor.
So in four years time, LLMs have gone from 1.5 billion parametres to 1 trillion parameters. GPT-5 might even be ready by 2023 according to some reports.
TL;DR: Read: 2023 State of AI in 14 Charts
Follow Jack Clark on Substack as well: in one of the most important Newsletters about A.I. called Import AI.

AI continued to post state-of-the-art results, but year-over-year improvement on many benchmarks continues to be marginal. Moreover, the speed at which benchmark saturation is being reached is increasing. However, new, more comprehensive benchmarking suites such as BIG-bench and HELM are being released.
Summaries
The new report shows several key trends in 2022:
AI continued to post state-of-the-art results on many benchmarks, but year-over-year improvements on several are marginal. Moreover, the speed at which benchmark saturation was being reached increased. Many traditional benchmarks, like ImageNet and SQuAD, that have been used to gauge AI progress no longer seem sufficient. New, more comprehensive benchmarking suites such as BIG-bench and HELM were released to challenge these increasingly capable AI systems.
Generative models such as DALL-E 2, Stable Diffusion, and ChatGPT became part of the zeitgeist. These showed impressive capability but raised ethical issues. Text-to-image generators are routinely biased along gender dimensions, and chatbots like ChatGPT can deliver misinformation or be used for nefarious purposes.
Large language models, which have driven much recent AI progress, are getting bigger and more expensive. For example, PaLM, one of the flagship models released in 2022, cost 160 times more and was 360 times larger than GPT-2 (just three years later), one of the first large language models launched in 2019.
AI is helping to accelerate scientific progress. In 2022, AI models were used to control hydrogen fusion, improve the efficiency of matrix manipulation, and generate new antibodies. AI has also started building better AI. Nvidia used an AI reinforcement learning agent to improve the design of the chips that power AI systems. Similarly, Google recently used one of its large language models, PaLM, to suggest ways to improve the very same model.
AI’s impressive technical progress has captured the attention of policymakers, industry leaders, and the public alike, although 2022 was the first time in a decade where AI investment levels cooled. More specifically:
An analysis of the legislative proceedings of 127 countries showed that the number of bills containing “artificial intelligence” passed into law grew from just 1 in 2016 to 37 in 2022. These laws ranged from mitigating the risks of AI-led automation to using AI for weather forecasting.
The proportion of companies adopting AI has plateaued over the past few years; however, the companies that have adopted AI continue to pull ahead. Companies that have embedded AI into their business offerings have realized both cost decreases and revenue increases. The AI capabilities most likely to be embedded by businesses are robotic process automation, computer vision, and virtual agents.
AI-related public opinion varies greatly by country. Chinese citizens feel much more positively about the benefits of AI products and services than Americans. Americans are excited about AI’s potential to make society better, save time, and improve efficiency but are concerned about labor automation, surveillance, and decreases in human connection.
For the first time in the last decade, year-over-year private investment in AI decreased. Global AI private investment was $91.9 billion in 2022, a 26.7% decrease from 2021. The total number of AI-related funding events as well as the number of newly funded AI companies likewise decreased. Still, AI private investment was 18 times greater than in 2013.
The total PDF runs 386 pages, I encourage you to take some time to go through it if you are a serious A.I. enthusiast.
The shear scope of how LLMs have scalted in number of parameters between 2019 and 2024 really blows me away. DeepMind (Google) said years ago how important that would be. I noticed a lot of the partners of HAI are Microsoft or Google affiliated.
I’ll have to continue this in another post, there’s a lot of unpack here. It’s the sixth edition of the AI Index Report HAI. Considering the hype, it’s a bit surprising investment was down in 2022, but rising interest rates is changing the venture capital reality across the board.